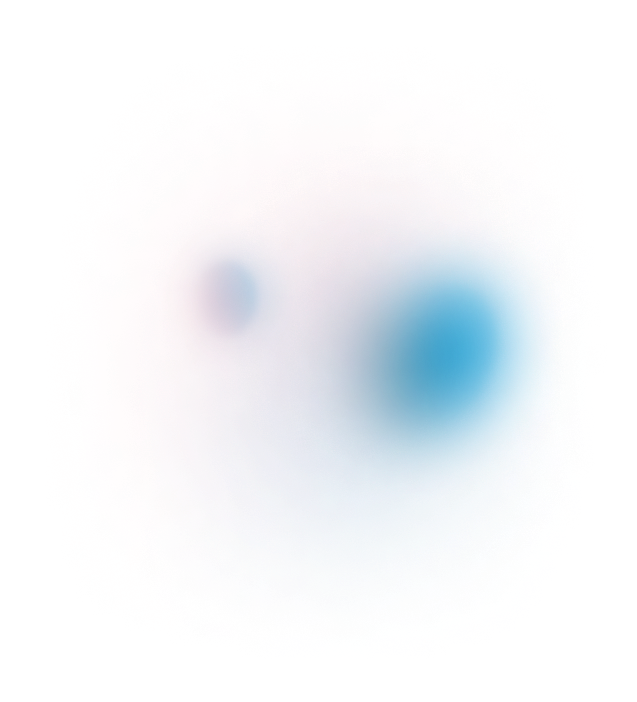
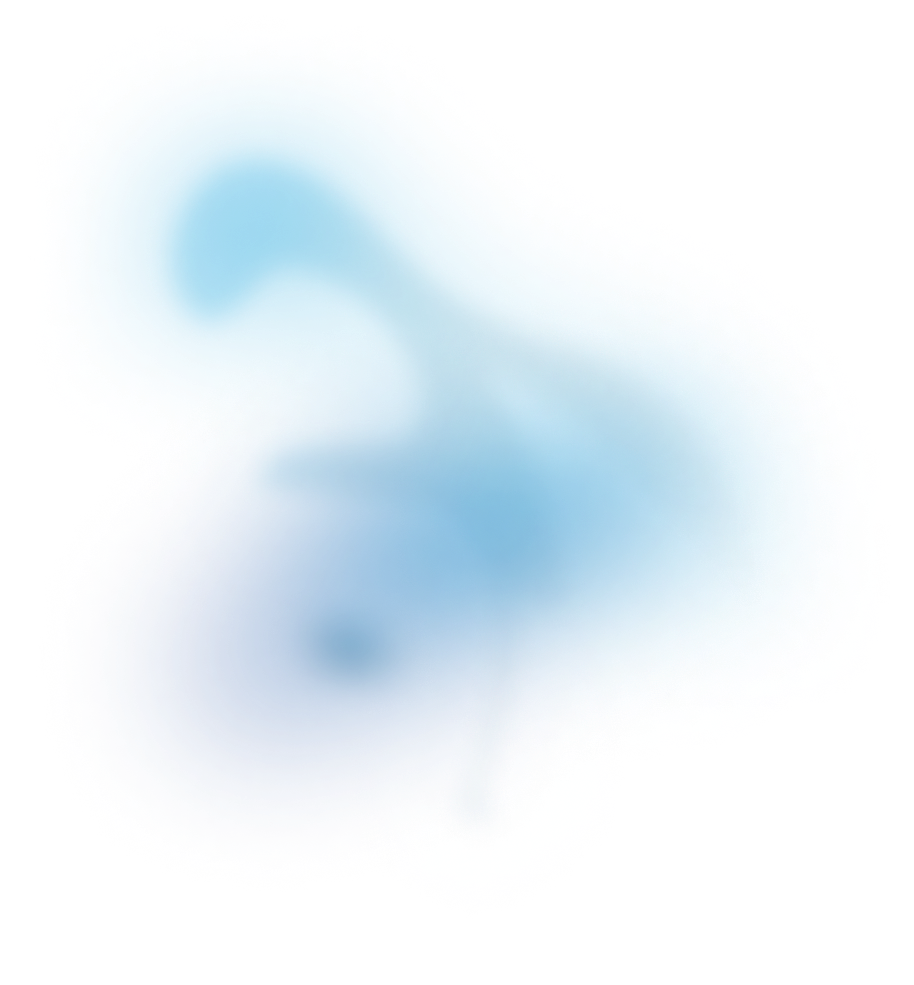
Unsupervised Machine Learning for Exploratory Data Analysis in Imaging Mass Spectrometry
In this review paper we give an extensive overview of the wide range of unsupervised machine learning methods that have been applied in the analysis of Mass Spectrometry Imaging (MSI) data.
Access publication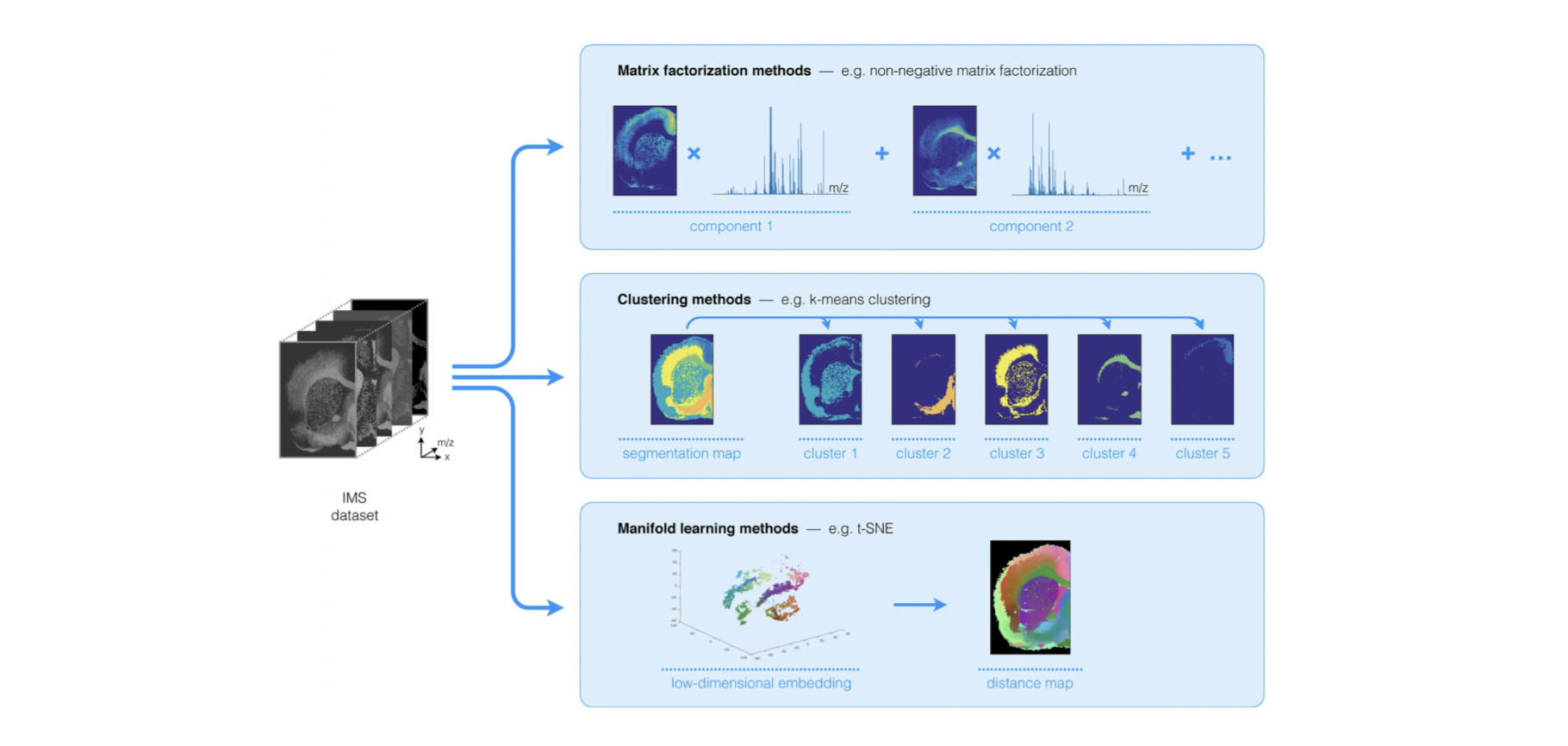
In this review paper we give an extensive overview of the wide range of unsupervised machine learning methods that have been applied in the analysis of Mass Spectrometry Imaging (MSI) data.
Unlike many other molecular imaging technologies, MSI does not require prior tagging of molecular targets and is able to measure large numbers of ions concurrently in a single experiment. While this makes the technology particularly suited for exploratory analysis, it also leads to very large and complex datasets (GB’s up to TB’s of raw data for a single experiment), making automated computational analysis indispensable.
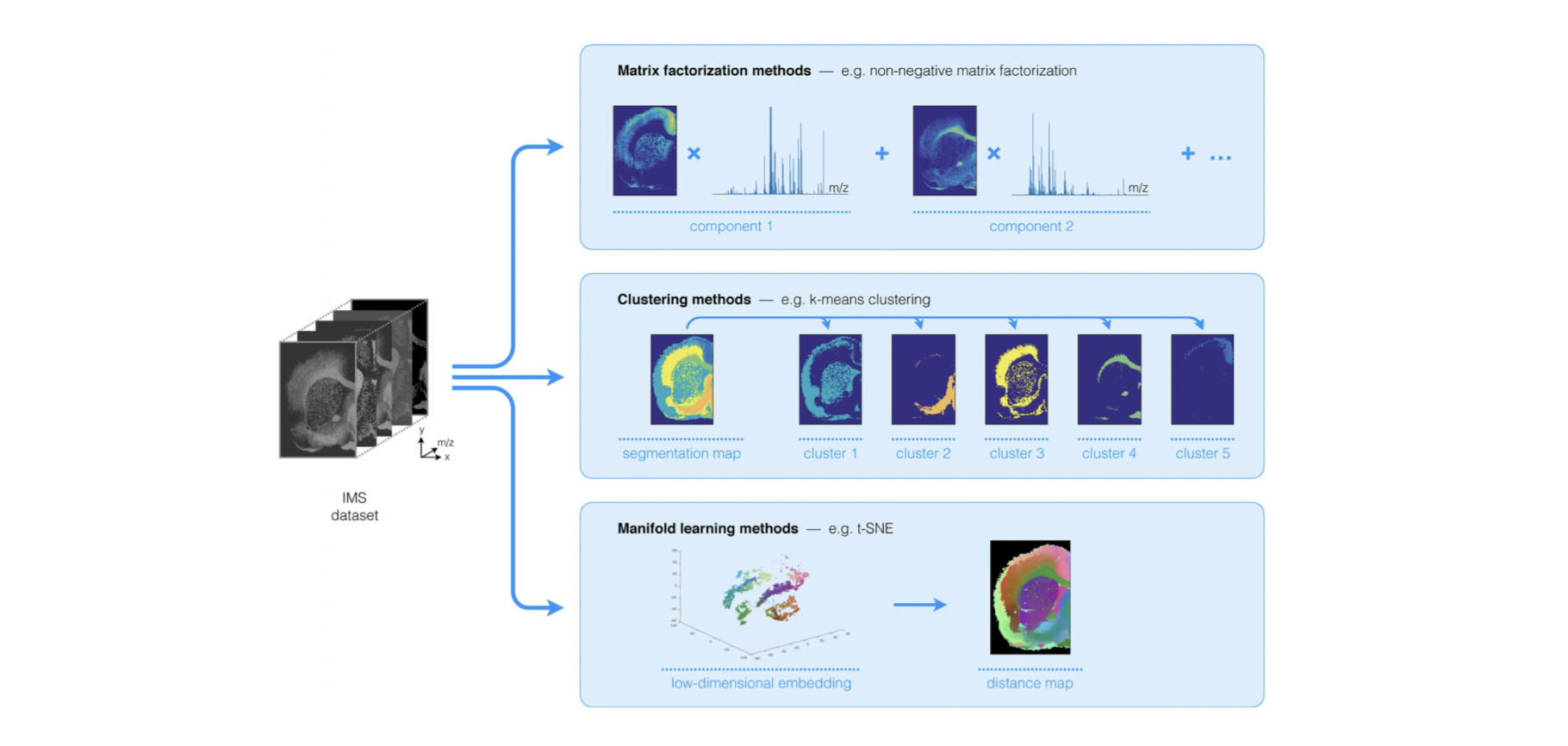
Unsupervised machine learning methods are primarily targeted at exploring the content of the data, and extracting their underlying trends in a mostly unbiased way. They are often the first step in gaining insight into a MSI dataset. A wide array of techniques has been used in the unsupervised analysis of MSI, which can broadly be broken down into 3 main categories, namely factorization methods, clustering methods and manifold learning or non-linear dimensionality reduction techniques. In this work we discuss the various machine learning methods for each class, and provide a theoretical basis for each method, along with their specific use cases in MSI applications.
This review aims to be an entry point for both (i) analytical chemists and mass spectrometry experts who want to explore computational techniques, and (ii) computer scientists and data mining specialists who want to enter the MSI field.
Publication details
Nico Verbeeck1,2,3, Richard M. Caprioli4,5,6,7,8, Raf Van de Plas1,4,5, Unsupervised Machine Learning for Exploratory Data Analysis in Imaging Mass Spectrometry, Mass Spectrometry Reviews 39:, 245–291, 2020