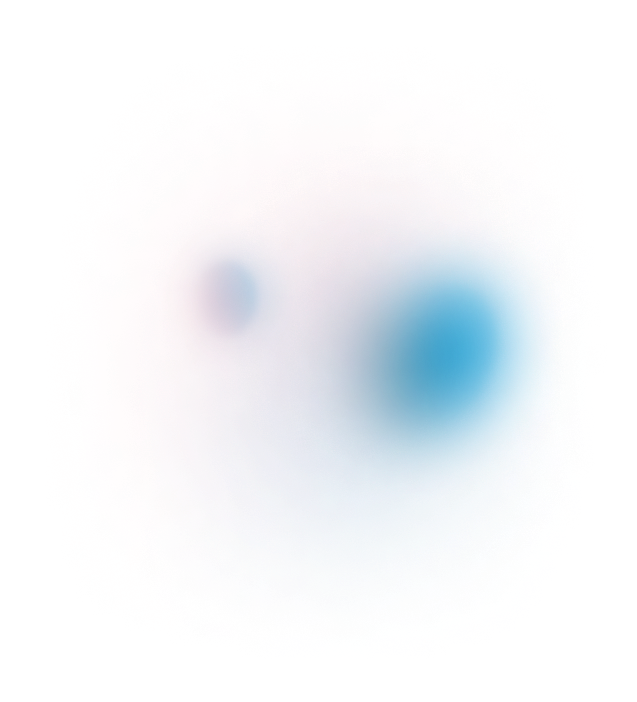
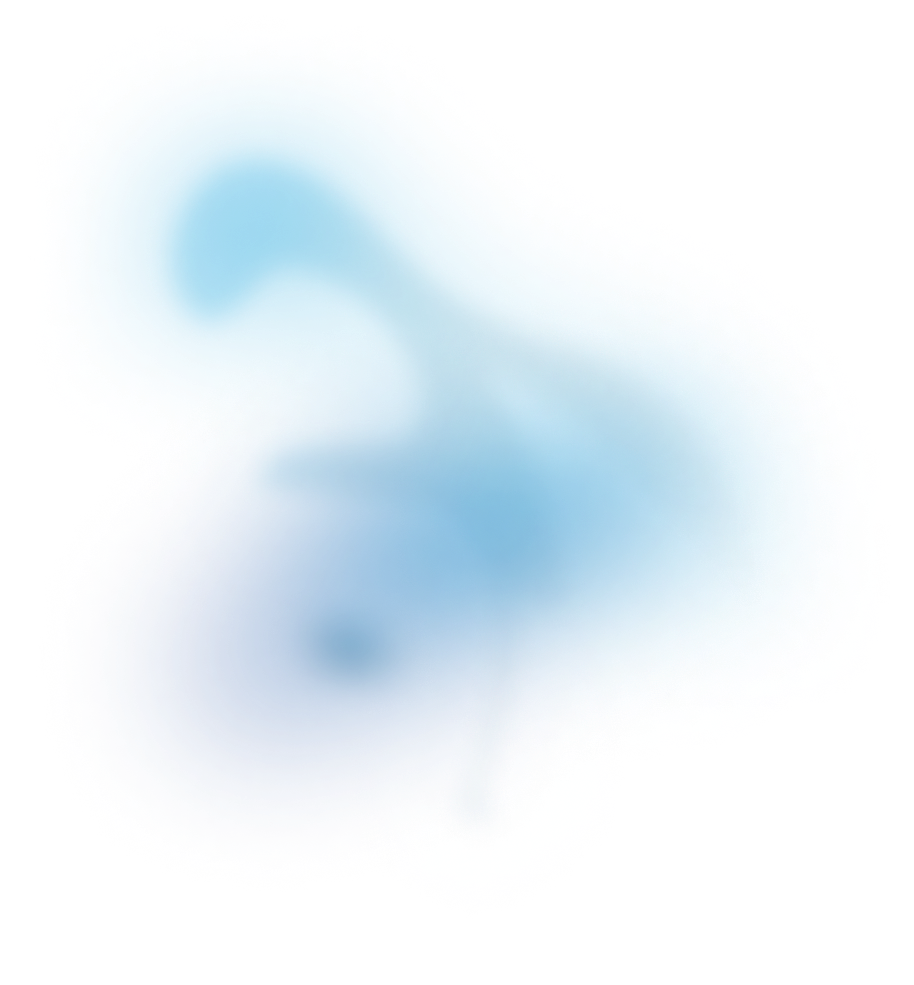
Evaluation of Distance Metrics and Spatial Autocorrelation in Uniform Manifold Approximation and Projection Applied to Mass Spectrometry Imaging Data
In this work, we explored the utility of a recently introduced, nonlinear dimensionality reduction method named Uniform Manifold Approximation (UMAP) for MSI data analysis.
Access publication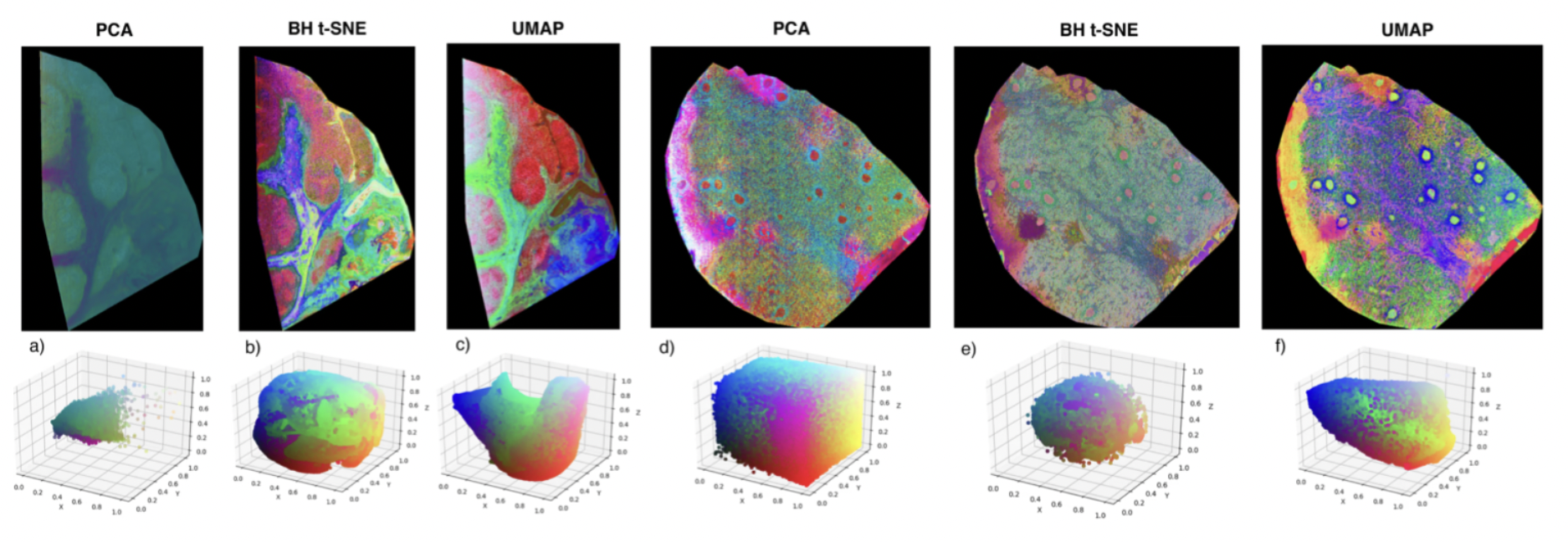
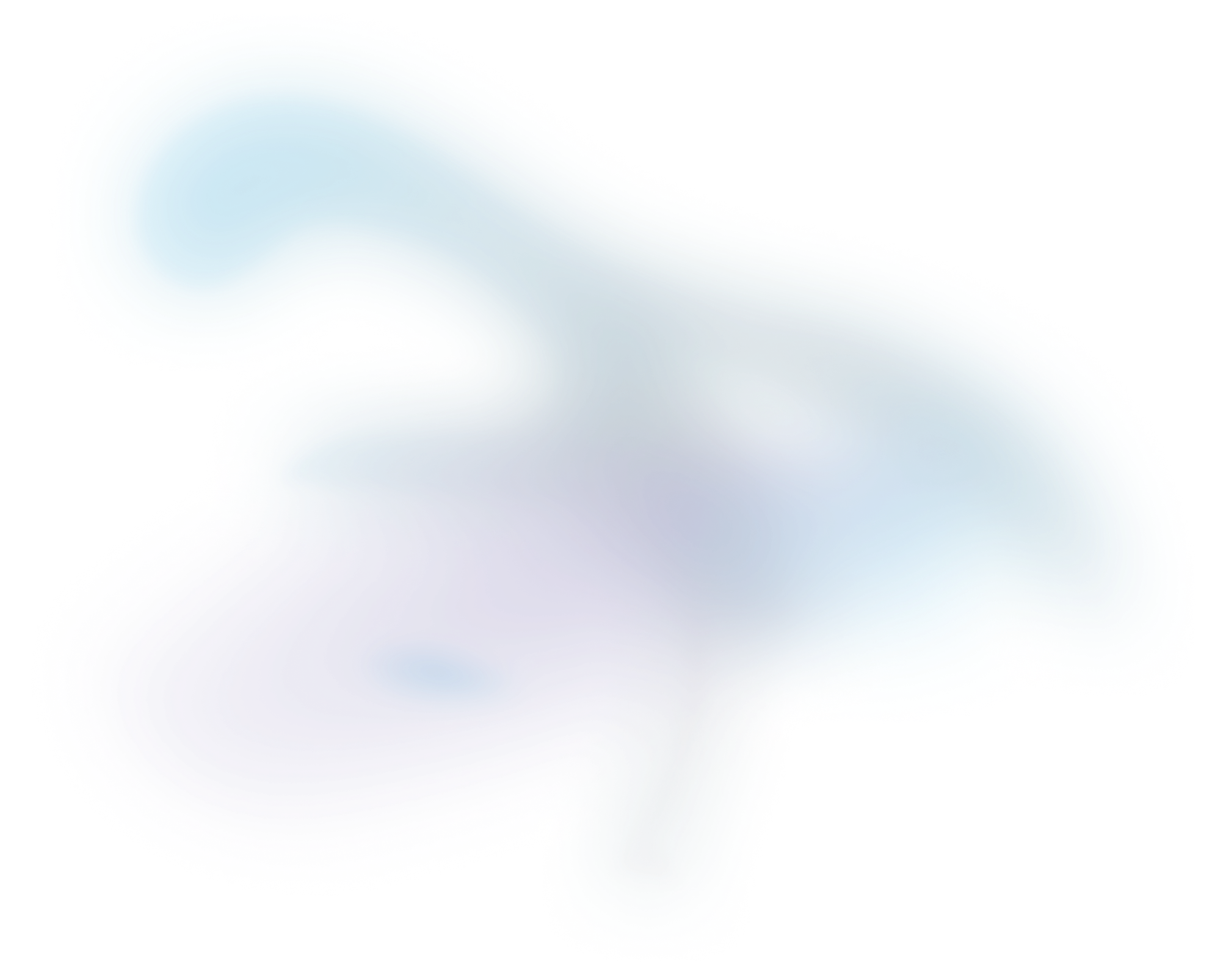
In this work, we explored the utility of a recently introduced, nonlinear dimensionality reduction method named Uniform Manifold Approximation (UMAP) for MSI data analysis. We compared UMAP to PCA and t-SNE. t-SNE is another pervasive nonlinear dimensionality reduction approach that is increasingly used for MSI data analysis. The work was primarily carried out by Tina Smets while receiving input and supervision from Nico Verbeeck and Marc Claesen for data analysis aspects.
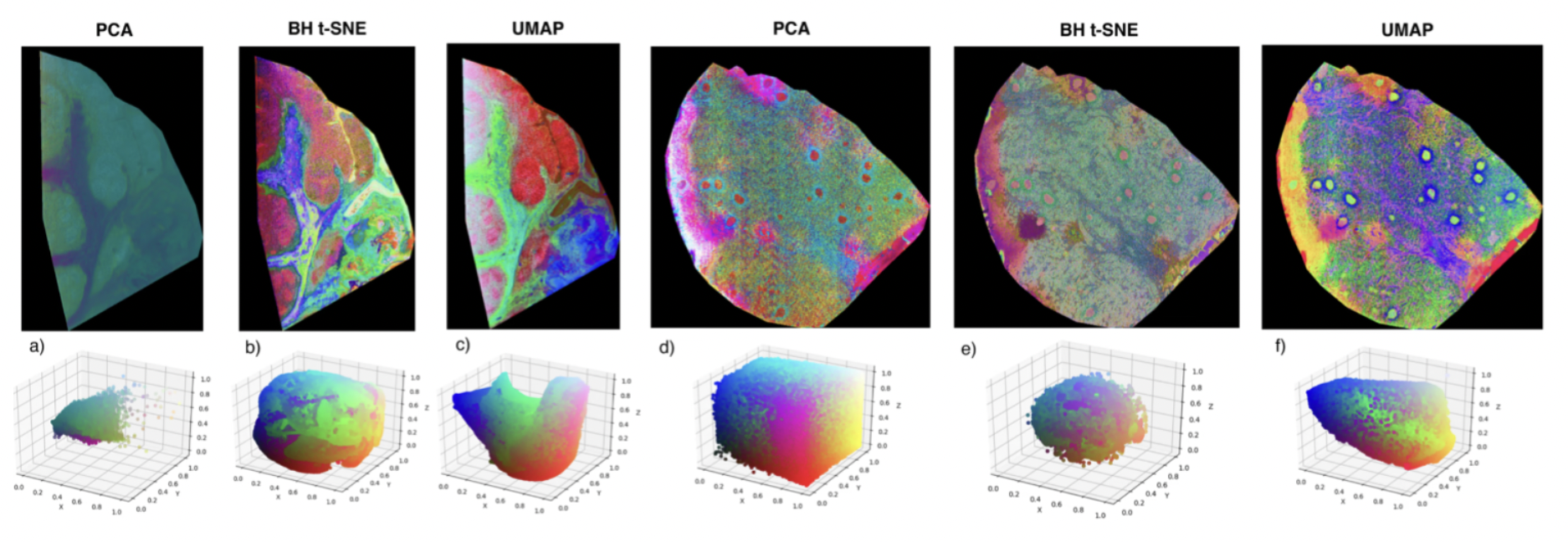
Specifically, our results illustrate that UMAP and t-SNE yield comparable results, which are clearly superior to simpler linear methods like PCA. Compared to t-SNE, however, UMAP provides significant computational advantages, namely:
- UMAP shows dramatically reduced computation time compared to t-SNE. In our experiments, we’ve observed an order of magnitude speedup of UMAP compared to the well-known Barnes-Hut approximation of t-SNE.
- In contrast to t-SNE, UMAP enables out-of-sample prediction, which means that the model can be used to embed data it was not trained on. This is a critical advantage for many applications.
Additional to the investigation of UMAP itself, we compared various distance metrics for MSI data. The results are shown in the figure below.
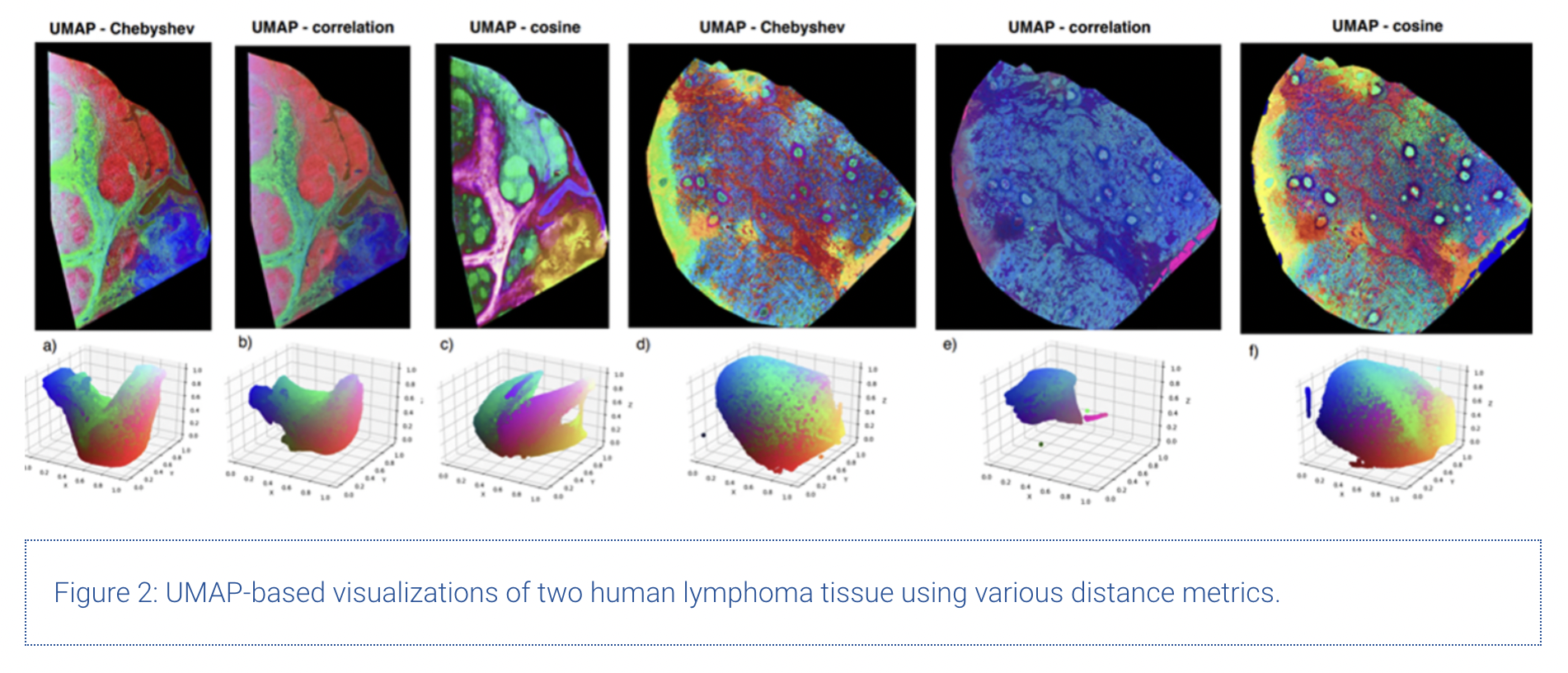
Upon comparing Figures 1 and 2, we can clearly see the superiority of distance metrics like cosine similarity and correlation compared to using standard Euclidian distance to model chemical similarity across spectra. The main underlying mathematical weaknesses of the Euclidian distance to model chemical similarity are its sensitivity to outliers (in this case m/z bins with very high intensity compared to the rest) along with its well-known problems when working in sparse, high-dimensional spaces.
Finally, during our investigation we identified a region of outlier pixels that skewed the UMAP analysis such that the dynamic range of colors was poorly used. After removing the impact of these outliers, we managed to improve our visualizations further.
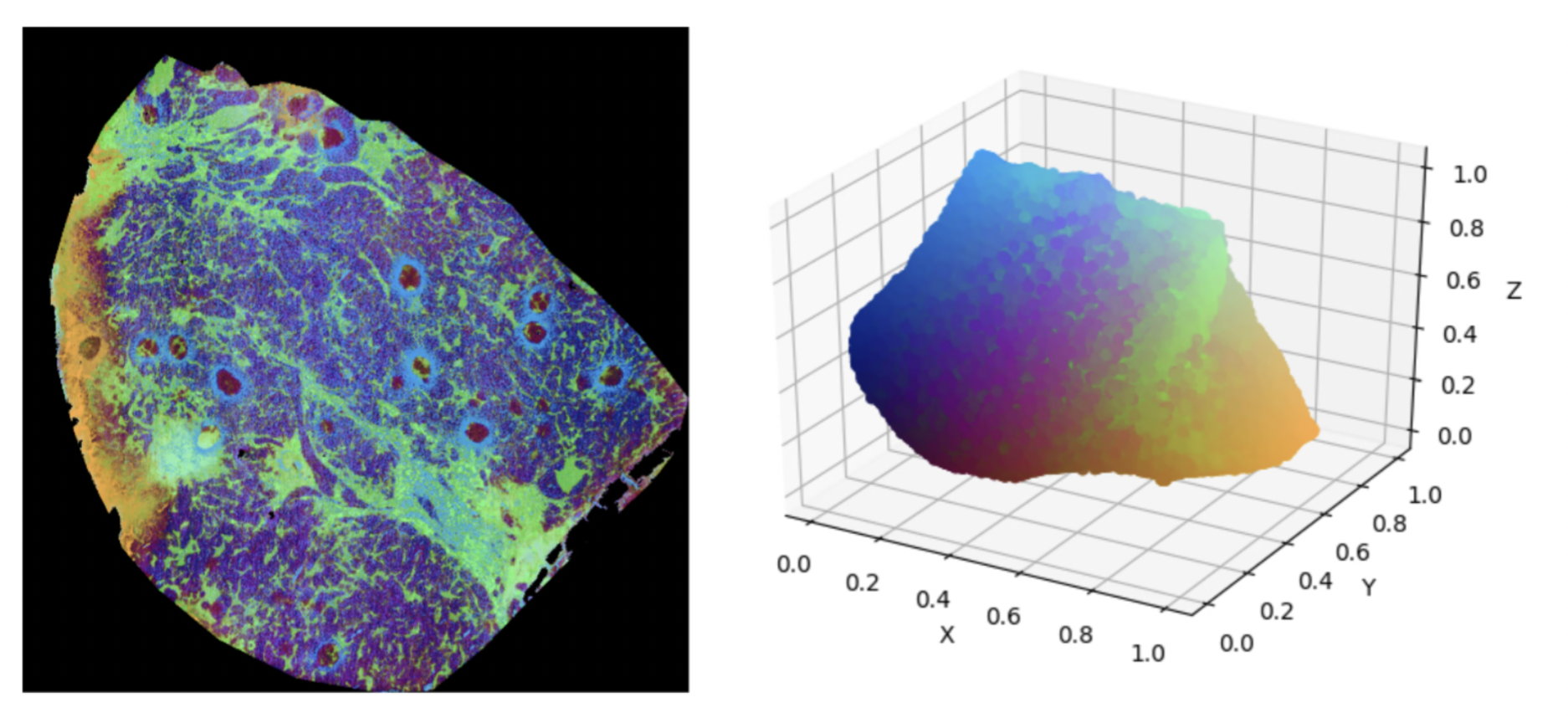